Py-ART Processing#
Overview#
Within this notebook, we will cover a few Py-ART processing routines:
Ground clutter detection
Attenuation correction
KDP estimation
Hydrometeor classification
# Imports
import numpy as np
import cartopy.crs as ccrs
import cartopy
import matplotlib.pyplot as plt
import pyart
pyart.config.load_config('../../pyrad_config/mf_config.py')
A module that was compiled using NumPy 1.x cannot be run in
NumPy 2.0.1 as it may crash. To support both 1.x and 2.x
versions of NumPy, modules must be compiled with NumPy 2.0.
Some module may need to rebuild instead e.g. with 'pybind11>=2.12'.
If you are a user of the module, the easiest solution will be to
downgrade to 'numpy<2' or try to upgrade the affected module.
We expect that some modules will need time to support NumPy 2.
Traceback (most recent call last): File "/srv/conda/envs/notebook/lib/python3.9/runpy.py", line 197, in _run_module_as_main
return _run_code(code, main_globals, None,
File "/srv/conda/envs/notebook/lib/python3.9/runpy.py", line 87, in _run_code
exec(code, run_globals)
File "/srv/conda/envs/notebook/lib/python3.9/site-packages/ipykernel_launcher.py", line 18, in <module>
app.launch_new_instance()
File "/srv/conda/envs/notebook/lib/python3.9/site-packages/traitlets/config/application.py", line 1075, in launch_instance
app.start()
File "/srv/conda/envs/notebook/lib/python3.9/site-packages/ipykernel/kernelapp.py", line 739, in start
self.io_loop.start()
File "/srv/conda/envs/notebook/lib/python3.9/site-packages/tornado/platform/asyncio.py", line 205, in start
self.asyncio_loop.run_forever()
File "/srv/conda/envs/notebook/lib/python3.9/asyncio/base_events.py", line 601, in run_forever
self._run_once()
File "/srv/conda/envs/notebook/lib/python3.9/asyncio/base_events.py", line 1905, in _run_once
handle._run()
File "/srv/conda/envs/notebook/lib/python3.9/asyncio/events.py", line 80, in _run
self._context.run(self._callback, *self._args)
File "/srv/conda/envs/notebook/lib/python3.9/site-packages/ipykernel/kernelbase.py", line 545, in dispatch_queue
await self.process_one()
File "/srv/conda/envs/notebook/lib/python3.9/site-packages/ipykernel/kernelbase.py", line 534, in process_one
await dispatch(*args)
File "/srv/conda/envs/notebook/lib/python3.9/site-packages/ipykernel/kernelbase.py", line 437, in dispatch_shell
await result
File "/srv/conda/envs/notebook/lib/python3.9/site-packages/ipykernel/ipkernel.py", line 362, in execute_request
await super().execute_request(stream, ident, parent)
File "/srv/conda/envs/notebook/lib/python3.9/site-packages/ipykernel/kernelbase.py", line 778, in execute_request
reply_content = await reply_content
File "/srv/conda/envs/notebook/lib/python3.9/site-packages/ipykernel/ipkernel.py", line 449, in do_execute
res = shell.run_cell(
File "/srv/conda/envs/notebook/lib/python3.9/site-packages/ipykernel/zmqshell.py", line 549, in run_cell
return super().run_cell(*args, **kwargs)
File "/srv/conda/envs/notebook/lib/python3.9/site-packages/IPython/core/interactiveshell.py", line 3048, in run_cell
result = self._run_cell(
File "/srv/conda/envs/notebook/lib/python3.9/site-packages/IPython/core/interactiveshell.py", line 3103, in _run_cell
result = runner(coro)
File "/srv/conda/envs/notebook/lib/python3.9/site-packages/IPython/core/async_helpers.py", line 129, in _pseudo_sync_runner
coro.send(None)
File "/srv/conda/envs/notebook/lib/python3.9/site-packages/IPython/core/interactiveshell.py", line 3308, in run_cell_async
has_raised = await self.run_ast_nodes(code_ast.body, cell_name,
File "/srv/conda/envs/notebook/lib/python3.9/site-packages/IPython/core/interactiveshell.py", line 3490, in run_ast_nodes
if await self.run_code(code, result, async_=asy):
File "/srv/conda/envs/notebook/lib/python3.9/site-packages/IPython/core/interactiveshell.py", line 3550, in run_code
exec(code_obj, self.user_global_ns, self.user_ns)
File "/tmp/ipykernel_348/282707559.py", line 8, in <module>
import pyart
File "/srv/conda/envs/notebook/lib/python3.9/site-packages/pyart/__init__.py", line 11, in <module>
from . import (
File "/srv/conda/envs/notebook/lib/python3.9/site-packages/pyart/aux_io/__init__.py", line 70, in <module>
from .pattern import read_pattern #noqa
File "/srv/conda/envs/notebook/lib/python3.9/site-packages/pyart/aux_io/pattern.py", line 23, in <module>
from ..core.radar import Radar
File "/srv/conda/envs/notebook/lib/python3.9/site-packages/pyart/core/__init__.py", line 40, in <module>
from .grid import Grid #noqa
File "/srv/conda/envs/notebook/lib/python3.9/site-packages/pyart/core/grid.py", line 26, in <module>
import xarray
File "/srv/conda/envs/notebook/lib/python3.9/site-packages/xarray/__init__.py", line 3, in <module>
from xarray import groupers, testing, tutorial
File "/srv/conda/envs/notebook/lib/python3.9/site-packages/xarray/groupers.py", line 15, in <module>
import pandas as pd
File "/srv/conda/envs/notebook/lib/python3.9/site-packages/pandas/__init__.py", line 26, in <module>
from pandas.compat import (
File "/srv/conda/envs/notebook/lib/python3.9/site-packages/pandas/compat/__init__.py", line 27, in <module>
from pandas.compat.pyarrow import (
File "/srv/conda/envs/notebook/lib/python3.9/site-packages/pandas/compat/pyarrow.py", line 8, in <module>
import pyarrow as pa
File "/srv/conda/envs/notebook/lib/python3.9/site-packages/pyarrow/__init__.py", line 65, in <module>
import pyarrow.lib as _lib
---------------------------------------------------------------------------
AttributeError Traceback (most recent call last)
AttributeError: _ARRAY_API not found
A module that was compiled using NumPy 1.x cannot be run in
NumPy 2.0.1 as it may crash. To support both 1.x and 2.x
versions of NumPy, modules must be compiled with NumPy 2.0.
Some module may need to rebuild instead e.g. with 'pybind11>=2.12'.
If you are a user of the module, the easiest solution will be to
downgrade to 'numpy<2' or try to upgrade the affected module.
We expect that some modules will need time to support NumPy 2.
Traceback (most recent call last): File "/srv/conda/envs/notebook/lib/python3.9/runpy.py", line 197, in _run_module_as_main
return _run_code(code, main_globals, None,
File "/srv/conda/envs/notebook/lib/python3.9/runpy.py", line 87, in _run_code
exec(code, run_globals)
File "/srv/conda/envs/notebook/lib/python3.9/site-packages/ipykernel_launcher.py", line 18, in <module>
app.launch_new_instance()
File "/srv/conda/envs/notebook/lib/python3.9/site-packages/traitlets/config/application.py", line 1075, in launch_instance
app.start()
File "/srv/conda/envs/notebook/lib/python3.9/site-packages/ipykernel/kernelapp.py", line 739, in start
self.io_loop.start()
File "/srv/conda/envs/notebook/lib/python3.9/site-packages/tornado/platform/asyncio.py", line 205, in start
self.asyncio_loop.run_forever()
File "/srv/conda/envs/notebook/lib/python3.9/asyncio/base_events.py", line 601, in run_forever
self._run_once()
File "/srv/conda/envs/notebook/lib/python3.9/asyncio/base_events.py", line 1905, in _run_once
handle._run()
File "/srv/conda/envs/notebook/lib/python3.9/asyncio/events.py", line 80, in _run
self._context.run(self._callback, *self._args)
File "/srv/conda/envs/notebook/lib/python3.9/site-packages/ipykernel/kernelbase.py", line 545, in dispatch_queue
await self.process_one()
File "/srv/conda/envs/notebook/lib/python3.9/site-packages/ipykernel/kernelbase.py", line 534, in process_one
await dispatch(*args)
File "/srv/conda/envs/notebook/lib/python3.9/site-packages/ipykernel/kernelbase.py", line 437, in dispatch_shell
await result
File "/srv/conda/envs/notebook/lib/python3.9/site-packages/ipykernel/ipkernel.py", line 362, in execute_request
await super().execute_request(stream, ident, parent)
File "/srv/conda/envs/notebook/lib/python3.9/site-packages/ipykernel/kernelbase.py", line 778, in execute_request
reply_content = await reply_content
File "/srv/conda/envs/notebook/lib/python3.9/site-packages/ipykernel/ipkernel.py", line 449, in do_execute
res = shell.run_cell(
File "/srv/conda/envs/notebook/lib/python3.9/site-packages/ipykernel/zmqshell.py", line 549, in run_cell
return super().run_cell(*args, **kwargs)
File "/srv/conda/envs/notebook/lib/python3.9/site-packages/IPython/core/interactiveshell.py", line 3048, in run_cell
result = self._run_cell(
File "/srv/conda/envs/notebook/lib/python3.9/site-packages/IPython/core/interactiveshell.py", line 3103, in _run_cell
result = runner(coro)
File "/srv/conda/envs/notebook/lib/python3.9/site-packages/IPython/core/async_helpers.py", line 129, in _pseudo_sync_runner
coro.send(None)
File "/srv/conda/envs/notebook/lib/python3.9/site-packages/IPython/core/interactiveshell.py", line 3308, in run_cell_async
has_raised = await self.run_ast_nodes(code_ast.body, cell_name,
File "/srv/conda/envs/notebook/lib/python3.9/site-packages/IPython/core/interactiveshell.py", line 3490, in run_ast_nodes
if await self.run_code(code, result, async_=asy):
File "/srv/conda/envs/notebook/lib/python3.9/site-packages/IPython/core/interactiveshell.py", line 3550, in run_code
exec(code_obj, self.user_global_ns, self.user_ns)
File "/tmp/ipykernel_348/282707559.py", line 8, in <module>
import pyart
File "/srv/conda/envs/notebook/lib/python3.9/site-packages/pyart/__init__.py", line 11, in <module>
from . import (
File "/srv/conda/envs/notebook/lib/python3.9/site-packages/pyart/aux_io/__init__.py", line 70, in <module>
from .pattern import read_pattern #noqa
File "/srv/conda/envs/notebook/lib/python3.9/site-packages/pyart/aux_io/pattern.py", line 23, in <module>
from ..core.radar import Radar
File "/srv/conda/envs/notebook/lib/python3.9/site-packages/pyart/core/__init__.py", line 40, in <module>
from .grid import Grid #noqa
File "/srv/conda/envs/notebook/lib/python3.9/site-packages/pyart/core/grid.py", line 26, in <module>
import xarray
File "/srv/conda/envs/notebook/lib/python3.9/site-packages/xarray/__init__.py", line 3, in <module>
from xarray import groupers, testing, tutorial
File "/srv/conda/envs/notebook/lib/python3.9/site-packages/xarray/groupers.py", line 15, in <module>
import pandas as pd
File "/srv/conda/envs/notebook/lib/python3.9/site-packages/pandas/__init__.py", line 49, in <module>
from pandas.core.api import (
File "/srv/conda/envs/notebook/lib/python3.9/site-packages/pandas/core/api.py", line 9, in <module>
from pandas.core.dtypes.dtypes import (
File "/srv/conda/envs/notebook/lib/python3.9/site-packages/pandas/core/dtypes/dtypes.py", line 24, in <module>
from pandas._libs import (
File "/srv/conda/envs/notebook/lib/python3.9/site-packages/pyarrow/__init__.py", line 65, in <module>
import pyarrow.lib as _lib
---------------------------------------------------------------------------
AttributeError Traceback (most recent call last)
AttributeError: _ARRAY_API not found
---------------------------------------------------------------------------
ValueError Traceback (most recent call last)
Cell In[1], line 8
5 import cartopy
6 import matplotlib.pyplot as plt
----> 8 import pyart
9 pyart.config.load_config('../../pyrad_config/mf_config.py')
File /srv/conda/envs/notebook/lib/python3.9/site-packages/pyart/__init__.py:11
7 from os import environ as _environ
9 # import subpackages
10 # print out helpful message if build fails or importing from source tree
---> 11 from . import (
12 __check_build, # noqa
13 aux_io, # noqa
14 bridge, # noqa
15 config, # noqa
16 core, # noqa
17 correct, # noqa
18 filters, # noqa
19 graph, # noqa
20 io, # noqa
21 map, # noqa
22 retrieve, # noqa
23 testing, # noqa
24 util, # noqa
25 )
26 from ._debug_info import _debug_info # noqa
28 # root level functions
File /srv/conda/envs/notebook/lib/python3.9/site-packages/pyart/aux_io/__init__.py:76
74 from .arm_vpt import read_kazr #noqa
75 from .edge_netcdf import read_edge_netcdf #noqa
---> 76 from .odim_h5 import read_odim_h5, read_odim_grid_h5, read_odim_vp_h5 #noqa
77 from .odim_h5_writer import write_odim_h5, write_odim_grid_h5 #noqa
78 from .gamic_hdf5 import read_gamic #noqa
File /srv/conda/envs/notebook/lib/python3.9/site-packages/pyart/aux_io/odim_h5.py:24
21 import numpy as np
23 try:
---> 24 import h5py
25 _H5PY_AVAILABLE = True
26 except ImportError:
File /srv/conda/envs/notebook/lib/python3.9/site-packages/h5py/__init__.py:25
19 # --- Library setup -----------------------------------------------------------
20
21 # When importing from the root of the unpacked tarball or git checkout,
22 # Python sees the "h5py" source directory and tries to load it, which fails.
23 # We tried working around this by using "package_dir" but that breaks Cython.
24 try:
---> 25 from . import _errors
26 except ImportError:
27 import os.path as _op
File h5py/_errors.pyx:1, in init h5py._errors()
ValueError: numpy.dtype size changed, may indicate binary incompatibility. Expected 96 from C header, got 88 from PyObject
Note that you can create your own Py-ART configuration file, which defines default field names, default colormaps, limits, and much more. This is the one we use at MeteoSwiss. You can then either load it at startup in your python code or define the environment variable PYART_CONFIG to point to your file in your work environment.
Reading the data#
Let’s start by loading our radar file which is of the standard CFRadial type. It corresponds to the third sweep of the operational radar scans, which is a PPI at 1° elevation. It contains raw radar data (before pre-processing) at a resolution of 83 m. We then add the temperature obtained from the COSMO NWP model to our radar object (note that this temperature was previously interpolated from the model grid to the radar polar grid). Note that the freezing level is quite high in this example (around 4200 m.).
# Open radar file
file_radar = './data/exercice1_swiss_thunderstorm/MHL2217907250U.003.nc'
radar = pyart.io.read_cfradial(file_radar)
# Add temperature
temp = pyart.io.read_cfradial('./data/exercice1_swiss_thunderstorm/20220628073500_savevol_COSMO_LOOKUP_TEMP.nc')
radar.add_field('temperature', temp.fields['temperature'])
Ground-clutter and noise removal#
Py-ART uses gatefilters which are a kind of mask to filter out problematic measurements. Most processing routines can take a gatefilter as input and will ignore pixels that were filtered out.
Here we create a gate filter based on the radar moments and their texture to filter out noise and ground clutter. Since we are interested in a strong thunderstorm we also extend this filter to remove all measurements with a SNR ratio of less than 10 dB.
gtfilter = pyart.filters.moment_and_texture_based_gate_filter(radar)
gtfilter.exclude_below('signal_to_noise_ratio', 10)
Let’s compare visually the reflectivity before and after filtering. Note that the plot function of Py-ART take a gatefilter as input.
fig, ax = plt.subplots(1,2, figsize=(10,6), sharex= True, sharey=True)
display = pyart.graph.RadarDisplay(radar)
display.plot_ppi('reflectivity', 0, vmin=0, vmax=60., ax = ax[0], colorbar_label = 'Raw')
display.plot_ppi('reflectivity', 0, vmin=0, vmax=60., gatefilter = gtfilter,
ax = ax[1], colorbar_label = 'Filtered')
ax[0].set_xlim([-50,50])
ax[0].set_ylim([-50,50])
ax[0].set_aspect('equal', 'box')
ax[1].set_aspect('equal', 'box')
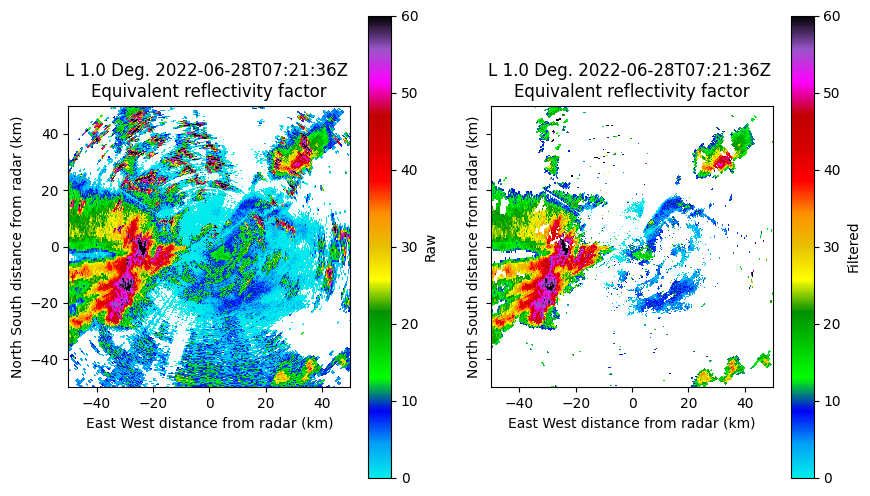
Here it is clear that most ground clutter (mostly north west and east of the radar), as well as noise have been filtered out.
Attenuation correction#
We can expect strong attenuation behind a thunderstorm like this. So it is a good idea to try to correct for it. Knowledge of the specific attenuation can also be very insightful.
out = pyart.correct.calculate_attenuation_zphi(radar, fzl = 4200,
gatefilter=gtfilter,
phidp_field = 'uncorrected_differential_phase',
temp_field = 'temperature',
temp_ref = 'temperature')
spec_at, pia, cor_z, spec_diff_at, pida, cor_zdr = out
radar.add_field('corrected_reflectivity', cor_z)
radar.add_field('corrected_differential_reflectivity', cor_zdr)
radar.add_field('specific_attenuation', spec_at)
Here we use the Z-PHI method, which uses the relation between differential phase shift and specific attenuation. However it works only in the liquid phase. So you need to provide it either with a fixed freezing level height, a field of freezing level heights or a field of temperature. Here we provide the later.
This method provides us with 5 output variables
specific attenuation dB/km
path integrated attenuation dB
corrected reflectivity dBZ
differential specific attenuation dB
path integrated differential attenuation dB
corrected differential reflectivity (ZDR) dB
We will now plot the specific attenuation as well as the raw and corrected reflectivities.
fig, ax = plt.subplots(1,3, figsize=(16,6), sharex= True, sharey=True)
display = pyart.graph.RadarDisplay(radar)
display.plot_ppi('specific_attenuation', 0, vmin=0, vmax=1.5, gatefilter = gtfilter,
ax = ax[0])
display.plot_ppi('reflectivity', 0, vmin=0, vmax=60., ax = ax[1], gatefilter = gtfilter,
colorbar_label = 'ZH with attenuation [dBZ]')
display.plot_ppi('corrected_reflectivity', 0, vmin=0, vmax=60., gatefilter = gtfilter,
ax = ax[2], colorbar_label = 'ZH attenuation corrected [dBZ]')
ax[0].set_xlim([-50,50])
ax[0].set_ylim([-50,50])
ax[0].set_aspect('equal', 'box')
ax[1].set_aspect('equal', 'box')
ax[2].set_aspect('equal', 'box')
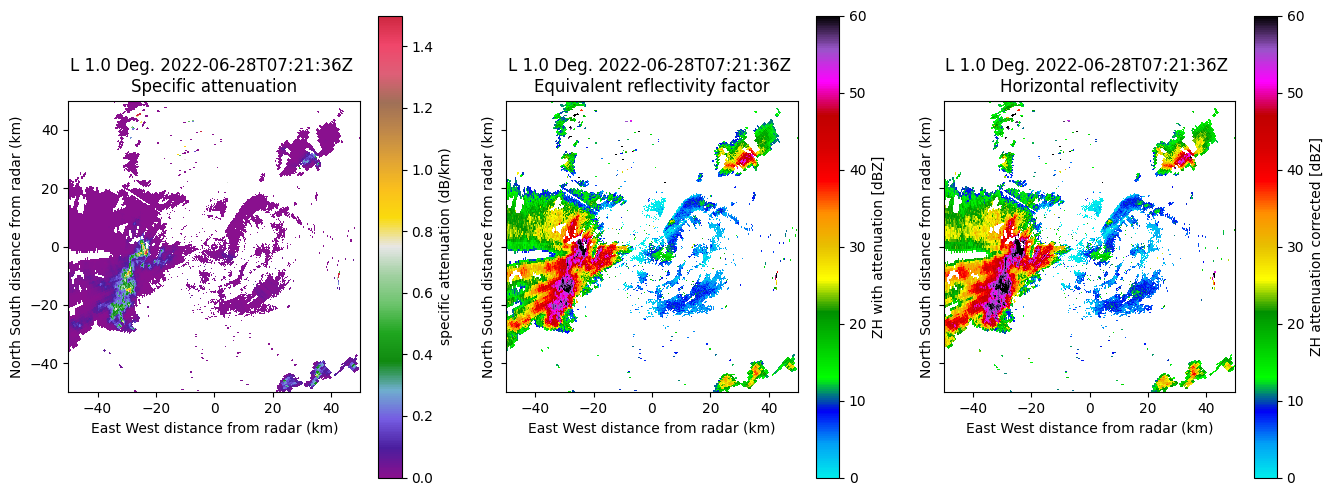
We can clearly observe a strong specific attenuation within the thunderstorm as well as a significant difference in reflectivity before/after correction behind the thunderstorm to the west.
KDP estimation#
Another very interesting radar variable is the specific differential phase shift KDP. Large KDP indicates the presence of large oblate drops and is linked to very strong precipitation. KDP is also needed for the hydrometeor classification algorithm. However KDP is not measured directly and needs to be estimated numerically from the raw differential phase shift (PHIDP). Py-ART provides three different retrieval methods. We will use the method by Maesaka et al. (2012) which is fast and robust but assumes KDP to be positive and is therefore limited to rainfall below the melting layer and/or warm clouds.
kdp, _, _ = pyart.retrieve.kdp_maesaka(radar, gatefilter = gtfilter,
psidp_field = 'uncorrected_differential_phase')
radar.add_field('specific_differential_phase', kdp)
fig, ax = plt.subplots(1,1, figsize=(6,6))
display = pyart.graph.RadarDisplay(radar)
display.plot_ppi('specific_differential_phase', 0, vmin = 0, vmax = 10,
ax = ax, gatefilter = gtfilter)
ax.set_xlim([-50,50])
ax.set_ylim([-50,50])
ax.set_aspect('equal', 'box')
/store/msrad/utils/anaconda3-wolfensb/envs/rainforest_tests/lib/python3.10/site-packages/numpy/core/fromnumeric.py:784: UserWarning: Warning: 'partition' will ignore the 'mask' of the MaskedArray.
a.partition(kth, axis=axis, kind=kind, order=order)
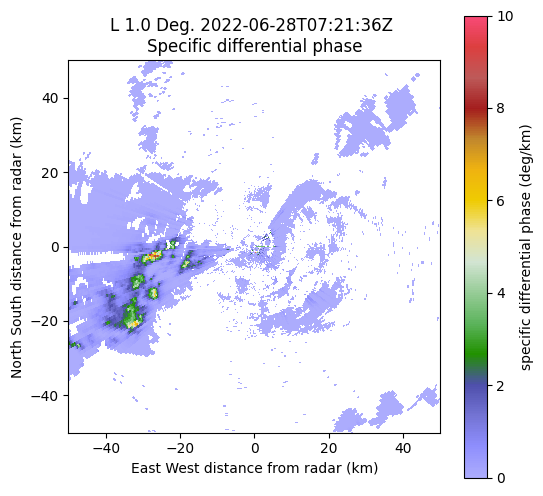
A look at the KDP field shows clusters of very large KDP (> 5 °/km) at the center of the thunderstorm.
Hydrometeor classification#
The hydrometeor classification algorithm in Py-ART by Besic et al. (2016) uses ZH, ZDR, RHOHV, KDP and the temperature to classify hydrometeors into one of 8 classes:
Ice hail, high density Graupel
Melting hail
Wet snow
Vertically oriented ice
Rain
Rimed particles
Light rain
Crystals
Aggregates
This algorithm requires centroids of polarimetric variables for the different hydrometeor classes. Below we provide it with centroids specifically suited for the radar of Monte Lema. If left empty, the algorithm will use default centroids at the right frequency band (X, C or S).
centroids = np.array([[13.8231,0.2514,0.0644,0.9861,1380.6],
[3.0239,0.1971,0.,0.9661,1464.1],
[4.9447,0.1142,0.,0.9787,-974.7],
[34.2450,0.5540,0.1459,0.9937,945.3],
[40.9432,1.0110,0.5141,0.9928,-993.5],
[3.5202,-0.3498,0.,0.9746,843.2],
[32.5287,0.9751,0.2640,0.9804,-55.5],
[52.6547,2.7054,2.5101,0.9765,-1114.6],
[46.4998,0.1978,0.6431,0.9845,1010.1]])
hydro = pyart.retrieve.hydroclass_semisupervised(radar, mass_centers = centroids,
refl_field = 'corrected_reflectivity',
zdr_field = 'corrected_differential_reflectivity',
kdp_field = 'specific_differential_phase',
rhv_field = 'uncorrected_cross_correlation_ratio',
temp_field = 'temperature')
radar.add_field('radar_echo_classification', hydro['hydro'])
fig, ax = plt.subplots(1,1, figsize=(6,6))
display = pyart.graph.RadarDisplay(radar)
import matplotlib as mpl
labels = ['NC','AG', 'CR', 'LR', 'RP', 'RN', 'VI', 'WS', 'MH', 'IH/HDG']
ticks = np.arange(len(labels)) + 1
boundaries = np.arange(0.5, len(labels)+1)
norm = mpl.colors.BoundaryNorm(boundaries, 256)
cax = display.plot_ppi('radar_echo_classification', 0, ax = ax, gatefilter = gtfilter,
norm = norm, ticks = ticks, ticklabs = labels)
ax.set_xlim([-50,50])
ax.set_ylim([-50,50])
ax.set_aspect('equal', 'box')
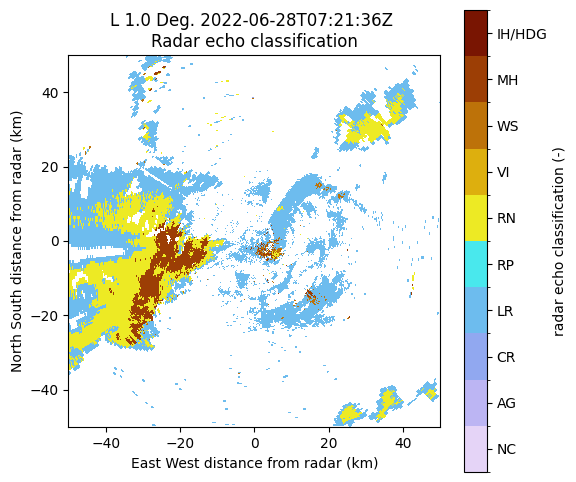
Note that the plotting commands are slightly more complicated due to the categorical colormap.
A look at the hydrometeor classification reveals the presence of wet hail in the center of the thunderstorm surrounded by rain and by light rain. A few isolated pixels (unfiltered ground clutter) are also classified as hail. There was indeed intense hail at the ground on that day.
QPE#
Py-ART provides several QPE algorithms but the most refined relies on the hydrometeor classification and uses different relations between radar variables and precipitation intensities within the different hydrometeor classes.
qpe = pyart.retrieve.est_rain_rate_hydro(radar, refl_field = 'corrected_reflectivity',
hydro_field = 'radar_echo_classification',
a_field = 'specific_attenuation',
thresh=40)
radar.add_field('radar_estimated_rain_rate', qpe)
We will now plot the precipitation intensity on a Cartopy map and add some spatial features (land borders) using RadarMapDisplay
lon_bnds = [8.2, 9.5]
lat_bnds = [45.5, 46.5]
display = pyart.graph.RadarMapDisplay(radar)
fig = plt.figure(figsize=(8,8))
display.plot_ppi_map('radar_estimated_rain_rate', 0, vmin=0, vmax=120.,
colorbar_label='', title='Precipitation intensity [mm/h]', gatefilter = gtfilter,
min_lon = lon_bnds[0], max_lon = lon_bnds[1],mask_outside = True,
min_lat = lat_bnds[0], max_lat = lat_bnds[1],
lon_lines=np.arange(lon_bnds[0], lon_bnds[1], .2), resolution='10m',
lat_lines=np.arange(lat_bnds[0], lat_bnds[1], .2),
lat_0=radar.latitude['data'][0],
lon_0=radar.longitude['data'][0], embellish=True)
states_provinces = cartopy.feature.NaturalEarthFeature(
category='cultural',
name='admin_0_countries',
scale='10m',
facecolor='none')
lakes = cartopy.feature.NaturalEarthFeature(
category='physical',
name='lakes',
scale='10m',
facecolor='blue')
rivers = cartopy.feature.NaturalEarthFeature(
category='physical',
name='rivers',
scale='10m',
facecolor='blue')
display.ax.add_feature(states_provinces, edgecolor='gray')
display.ax.add_feature(lakes, edgecolor='blue', alpha = 0.25)
display.ax.add_feature(cartopy.feature.RIVERS)
/users/wolfensb/pyrad/src/pyart/pyart/graph/radarmapdisplay.py:287: UserWarning: No projection was defined for the axes. Overridding defined axes and using default axes with projection Lambert Conformal.
warnings.warn(
<cartopy.mpl.feature_artist.FeatureArtist at 0x2af9b0230280>
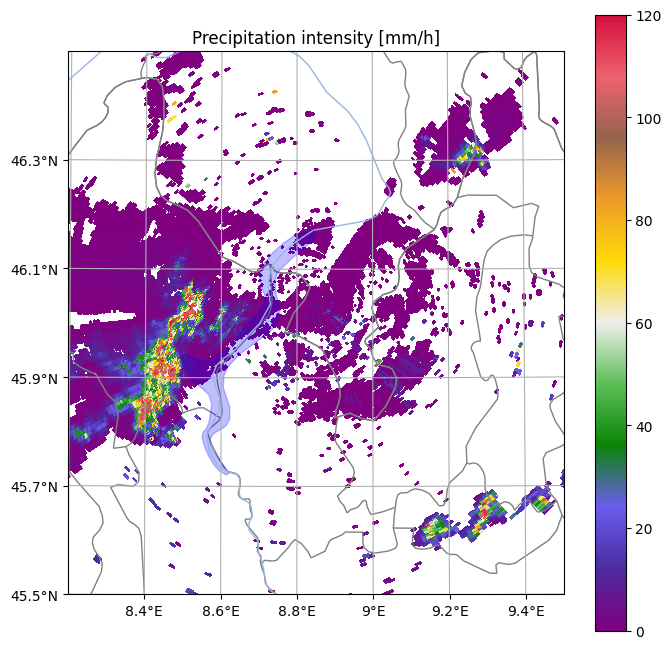
Note that we didn’t estimate precipitation intensity at the ground but only aloft. Within the thunderstorm precipitation intensity is extremely high. This is likely too high because QPE in wet hail is very uncertain. However, even the operational QPE algorithm at MeteoSwiss estimated precipitation intensities at the ground close to 120 mm/h.
Resources and References#
MeteoSwiss Py-ART essentials links: